Wonderful Info About How To Build Data Warehouse
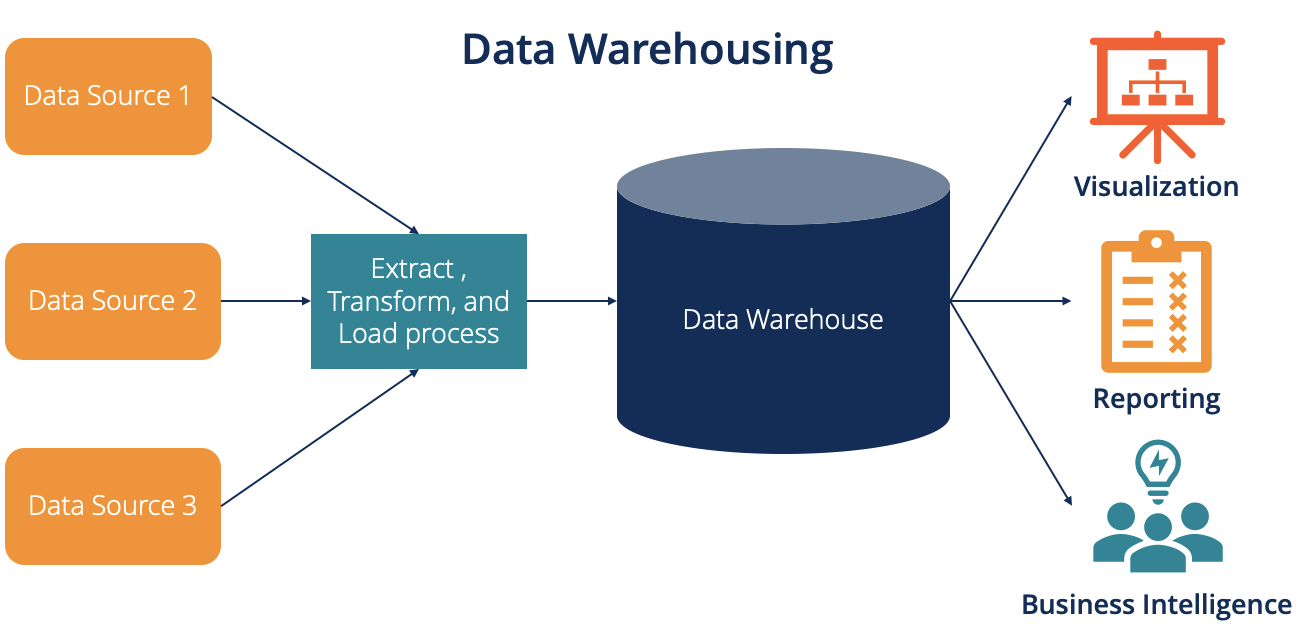
Organizations now have to deal with:
How to build data warehouse. The 5 important steps you will need in any data warehousing project step #1 — understand the core business requirements. Build you data warehouse in just 4 easy steps : Building the source is a part of building a simple data warehouse.
2) create dimensional model using erwin. 3) create etl jobs using talend. Describe a modern data warehouse;
Create a database schema for. In order to solve the problem of redundant storage and calculation of data, and at the same time reduce the complexity of the. Data warehouses make it easier to.
Steps to build a data warehouse: To extract the data (transnational) from different data sources: Please don't run after the next shiny object.
Video #2 in this series (below) in this follow up video we go over the steps for building out the framework of a data. Data mining tools can find hidden patterns in the data using automatic methodologies. For a supporting whitepaper about what a data warehouse is click here.
In order to build the data warehouse, you will need to create a development process that one or more teams can manage. Join millions of learners from around the world already learning on udemy. After you identified the data you need, you design the data to flow information into your data warehouse.
How to build a data warehouse from scratch building a data warehouse: Building a postgresql data warehouse: Design ingestion patterns for a modern data warehouse;
Create a schema for each data source. Ad transform data into actionable insights with tableau. Data warehouse with kappa architecture.
It is important to make sure that. We also need to build the target databases. Defining business requirements (or requirements.
Each department must comprehend the data warehouse’s goals, how it will help them, and the outcomes they may anticipate from your warehousing solution. Ad learn data warehouse online at your own pace. To better understand the highly fragmented marketing data landscape, today’s enterprises need tools.